Earth – a marvelous blue marble in the vastness of space is the only known place in the Universe that supports life. Our planet has witnessed multiple phases, of destruction and restoration alike, and has emerged triumphant on numerous occasions. But, at present, we stand at the cusp of an unimaginable and unpredictable future, and we, the most intelligent species ever to roam on the planet’s surface, are to blame for it.
Our planet’s ecosystem is a complex and interwoven system comprising all living organisms and their physical surroundings. Made up of various biotic (plants, animals, and microorganisms) and abiotic components (atmosphere, hydrosphere, geosphere, and cryosphere) that interact with each other, the ecosystem creates and sustains conditions that support life on Earth.
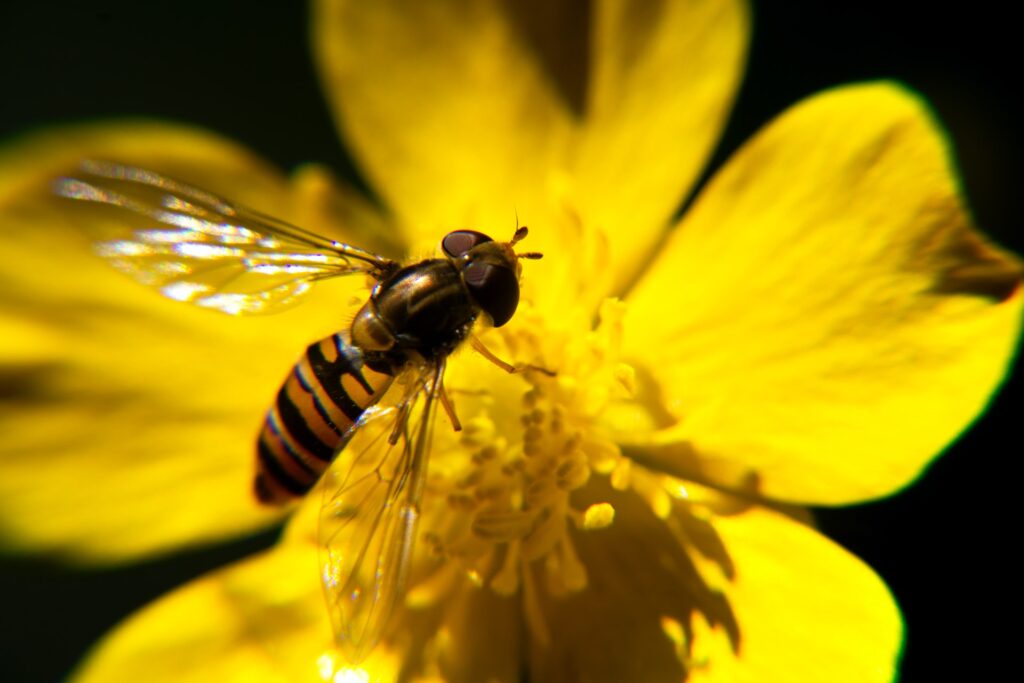
Due to recent human activity, the sustenance component of the ecosystem hangs in balance. Especially after the Industrial Revolution, the impact of humans on Earth’s resources and biodiversity is many-folds.
What is Sustainability?
Sustainability refers to the ability to meet the needs of the present without compromising the ability of future generations to meet their own needs. The concept of sustainability aptly includes environmental, economic, and social dimensions. In practice, sustainability efforts focus on reducing negative impacts on the environment and promoting economic growth that is inclusive and equitable.
Sustainability plays a crucial role in Earth’s ecosystem as it ensures that its resources are utilized in a way that preserves its ability to support life in the future. Without a practice of sustainability, the ecosystem would be at risk of degradation as disruptions to one part of the system can have ripple effects throughout the system.
Sustainability is also vital for addressing climate change, one of the most pressing issues at hand. Mostly caused by human activities that result in the release of greenhouse gases into the atmosphere, climate change is altering the planet’s life-generating cycle as a whole.
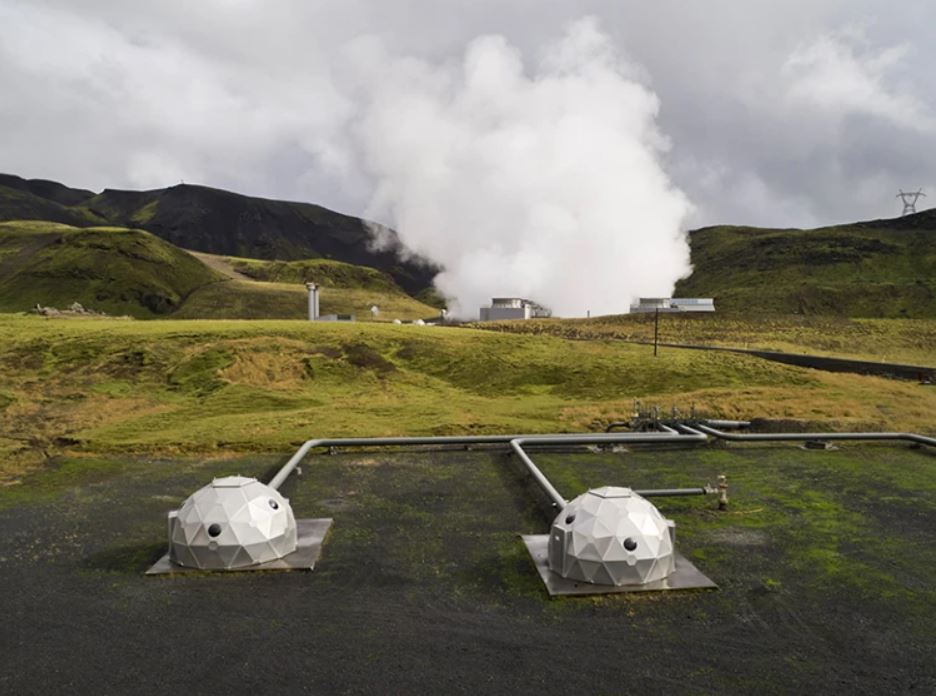
How can Artificial Intelligence (AI) help?
Artificial Intelligence has the potential to play a significant role in supporting sustainable development. AI can help in optimizing and automating processes, improving decision-making, and increasing efficiency, thereby helping to reduce negative impacts on the environment.
AI-powered algorithms can monitor and control energy usage in real-time and make adjustments as needed to minimize energy waste. AI can also aid in improving rural and urban planning and management, reducing unrequired resource allocations, and improving the liveability of cities.
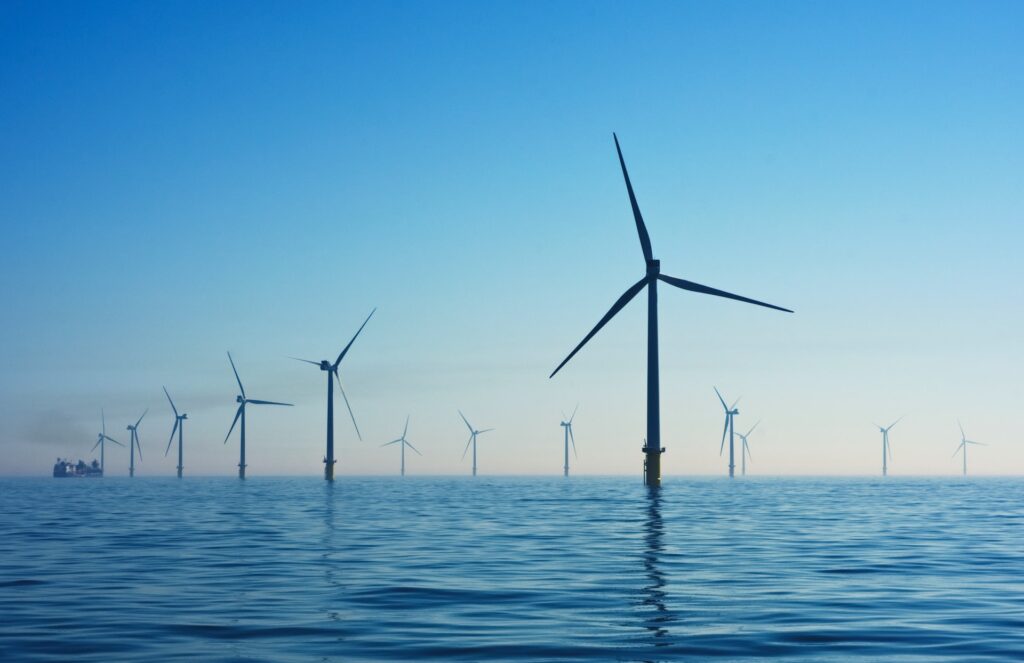
In agriculture, AI-based models can optimize crop yields, reduce water consumption and fertilizer use, and improve soil health. By analyzing data on weather, soil conditions, and crop growth, AI-powered systems can predict crop yields with enhanced accuracy.
In transportation and logistics, AI algorithms can analyze traffic data, delivery schedules, and weather data to predict and identify the most optimized routes, thereby reducing fuel consumption and making logistics more sustainable.
Below is a case study elaborating on the use of artificial intelligence in satellite imagery to predict and map populations in rural India which otherwise was missing in the census data.
Case Study: Mapping Missing Population in Rural India by Cornell University [1]
As per recent estimates, around 300-350 million people worldwide are not reflected in their respective country’s census data. This adversely impacts the ability to correctly measure any progress on the SDG front. For example, an inaccurate population distribution would lead to an inaccurate policy framework by the government to cater to the region’s socio-economic statistics. Another example can be related to sending help in case of natural disasters such as floods and earthquakes – if population mapping is correct, rescue efforts and other help can be organized much more efficiently.
The traditional method of creating a population distribution map is done by performing a national census which is usually expensive. In some countries, this activity is carried out once in a decade, or once in a couple of decades. So, relying on the older census data can be prone to errors if any policy has to be devised or any resource allocation is needed urgently.
Researchers at Cornell University took a different approach to tackling this issue. They utilized data from high-resolution satellite images and fed it to a machine-learning model to predict the population density of rural India.
The input comprised datasets from satellite images of Landsat-8 and Sentinel-1 satellites. Landsat-8 is a collaboration between NASA and the USGS (U.S. Geological Survey) for providing measurements of our planet’s terrestrial and polar regions. Sentinel-1 is a radar-imaging satellite, so it can capture images regardless of the weather, and can accurately capture roads and roofs based on the ground surface reflectance.
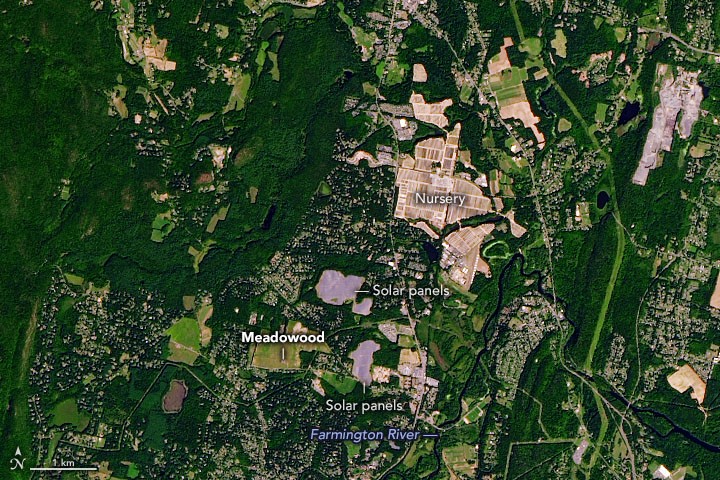
The researchers proposed a deep-learning approach by feeding the satellite images as inputs to a CNN (Convolutional Neural Network) model. A CNN is a kind of deep learning model that can process data having grid patterns, such as images, and is designed to adaptively learn spatial features, ranging from low-level to high-level patterns. With the help of a technique called ‘Convolution’, a CNN model can convert the images to a form that is easier to process without compromising on the features critical for making a good prediction.

Note: This paragraph along with the preceding image can be skipped by people belonging to non-technical specialties.
Two custom CNN architectures were designed as shown in the above image. The first architecture, known as the Shallow Combo is a modified version of the VGG16 architecture (a CNN model supporting 16 layers). Images from Landsat and Sentinel-1 are input separately as two branches which are concatenated along the channel dimension. The arrangement undergoes a 1X1 convolution filter, the output of which becomes the input for the rest of the VGG16 layers. In the last step, one more fully connected layer of size 100 is added to the network in order to predict the output log to the base 2 population density. The second architecture called Deep Combo is a modified version of the former in which the images are fed through all the convolutional layers first before applying the 1X1 convolution filter. Shallow Combo performs better for predicting population of a single village, whereas Deep Combo is suitable for predicting aggregated population at a subdistrict level.
The researchers first trained the model on a single state (Gujarat) and then scaled it up to train on around 3,50,000 villages in all the states of India. The evaluation metrics were mainly based on two statistical parameters – R^2 and Pearson Correlation. While R^2 measures the true variance in a model and attains a perfect value at 1, Pearson Correlation measures the linear correlation between the predicted and actual values and ranges from -1 to +1.
The model was able to predict aggregated village population in one subdistrict with an R^2 of 0.93 (the closer to 1 the better) and a Pearson Correlation value of 0.96 (again a better prediction).
Such models can likely be useful for governments to plan better resource allocations in times of natural disasters, cater to food, water, and energy demands appropriately, aid in disease tracking efficiently, and thus measure progress remarkably for the SDGs.
Conclusion
In conclusion, the ability of AI to learn, predict, and assist humans in decision-making will be a powerful tool in sustainability efforts. However, it is worth mentioning that the development, creation, and use of AI must be just in itself, taking into account ethical and societal implications.
Notes:
- Mapping Missing Population in Rural India: A Deep Learning Approach with Satellite Imagery https://doi.org/10.48550/arXiv.1905.02196
- How one of the world’s largest wind companies is using AI to capture more energy https://news.microsoft.com/europe/features/winds-of-change-how-one-of-the-worlds-largest-wind-companies-is-using-ai-to-capture-more-energy/
Kindly subscribe to our newsletter to be informed about the articles as soon as they are published.
This article is authored by Abhinav Singh.
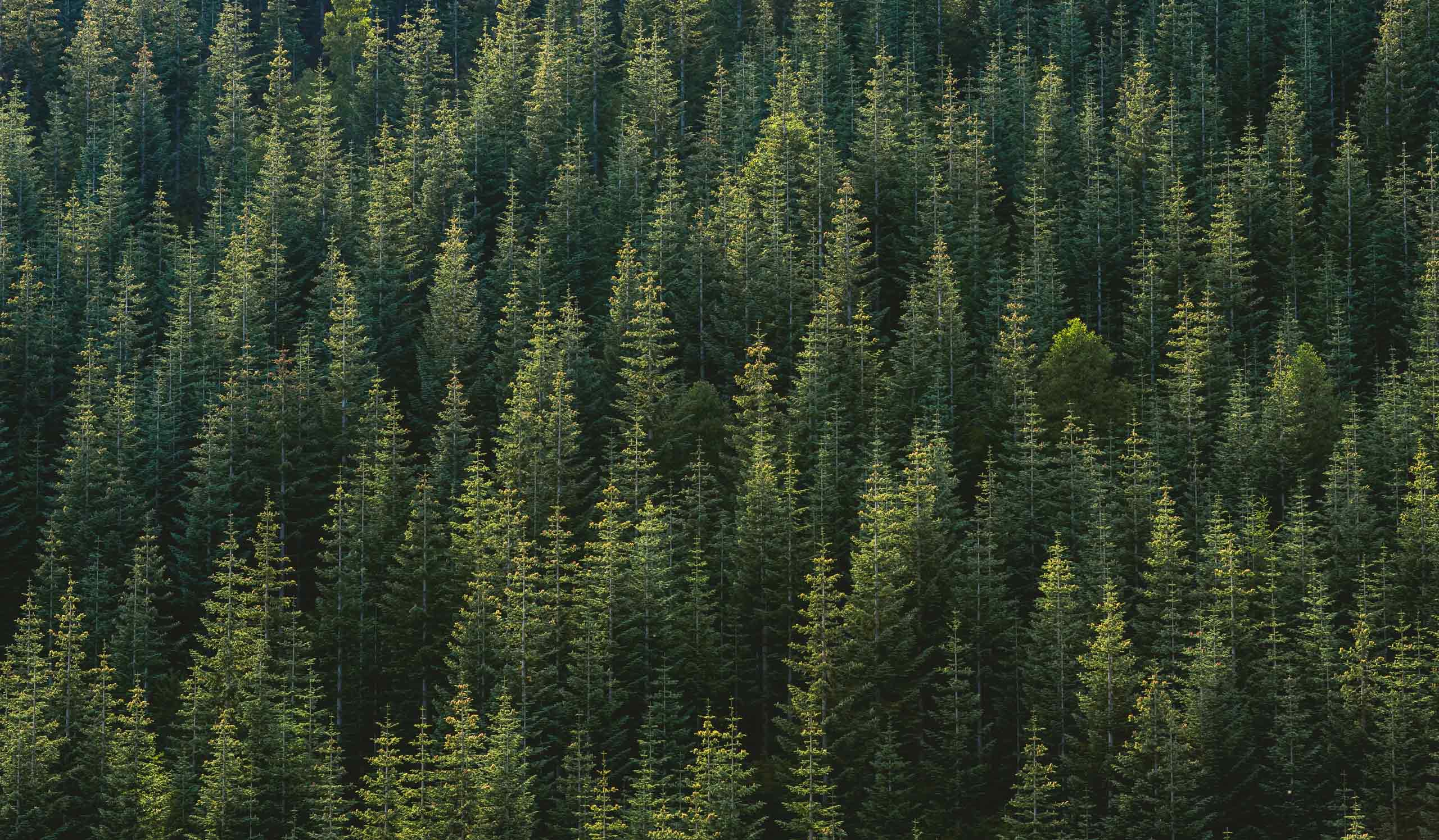
Subscribe to Primitive Proton Newsletter
Sign up to keep up to date with the latest news and curated blogs in the world of space, science, and technology.
Your email is never shared with anyone. You can opt out anytime with a simple click!
WE PRIORITISE PRIVACY.