Earth as an ecosystem is so much diverse and yet so complex. The beauty lies in the intricate balance of all the constituents thriving within it. From the tallest trees to the smallest creatures, each species play a vital role in maintaining balance and contributing to its stunning natural beauty.
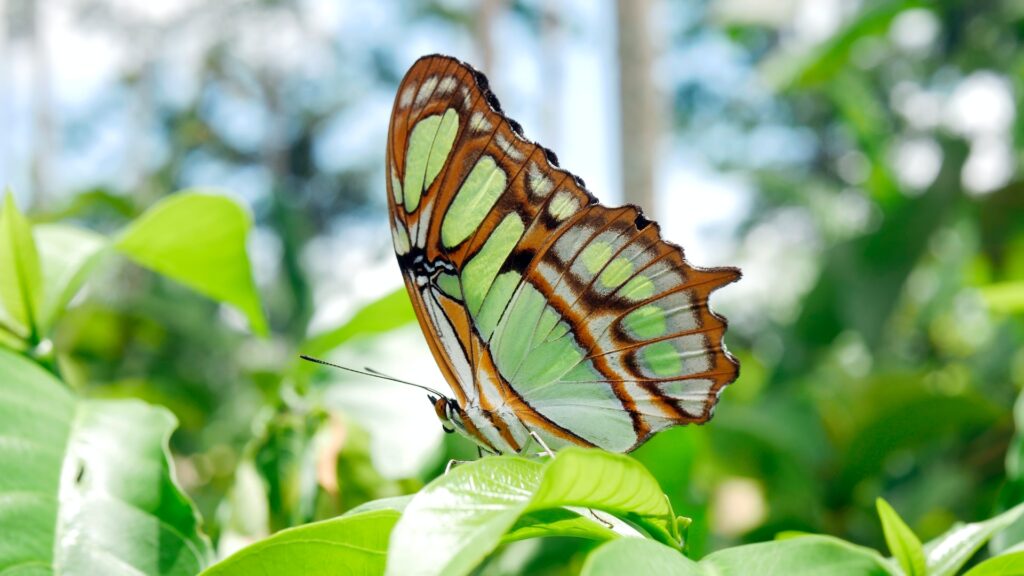
The ecological balance that our planet has been able to maintain for millennia is no longer safe courtesy of the widespread climate changes. Human activities such as deforestation, inappropriate ways of land use, and fossil fuel consumption are contributing to an exponential increase in greenhouse gas emissions, thereby significantly changing Earth’s climate and biodiversity.

Ecosystem sustainability is the need of the hour. A balanced ecosystem helps in preserving the flora and fauna and supports the livelihood of millions of people, especially those who depend on hunting, fishing, and agriculture.
The United Nations Sustainable Development Goals (SDGs) are a set of 17 identified goals as part of the 2030 Agenda for Sustainable Development. The SDGs act as a universal call and reminder to end poverty, conserve and protect the planet, and ensure everyone enjoys harmony and prosperity.
In order to provide a toolkit for research professionals to carry out multi-disciplinary analysis, the Shared Socioeconomic Pathways (SSPs) were developed in collaboration among climate scientists, economists, and energy systems modelers.

Forests are one of the vital components of the ecosystem, especially the global Carbon Cycle (the Carbon Cycle is a process of movement of carbon from land and water bodies through the atmosphere and living organisms and is fundamental to life on Earth).
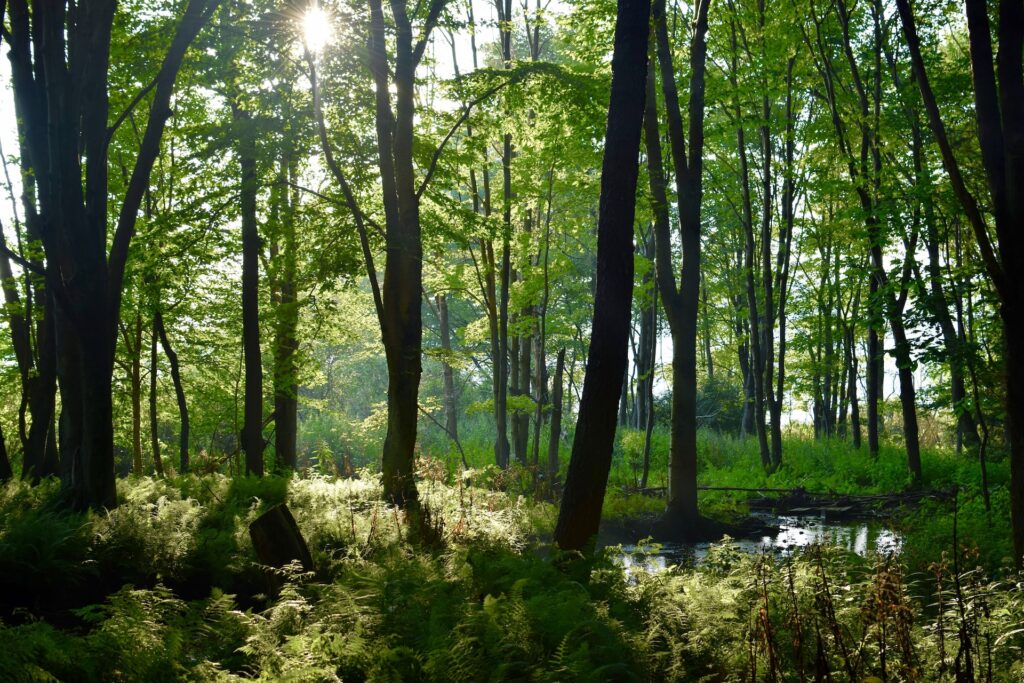
As per the estimates, forests around the world absorb about 7.6 billion metric tons of carbon dioxide per year and act as a huge carbon sink (a forest is said to be a carbon sink if it absorbs more carbon than it releases, and a carbon source if it releases more carbon than it absorbs).
But with the advance in factors such as deforestation, fires, droughts, insect outbreaks, etc., the trend seems to be reversing. For example, forests in Canada have become carbon sources – they are releasing more carbon than they are sequestering from the atmosphere.

Case Study: Predicting Climate-Sensitive Risks to Forests by CarbonPlan
Researchers at CarbonPlan, a non-profit public benefit corporation based out of California, carried out a collaborative study to predict increases in climate-sensitive risks in the Western U.S. throughout the 21st century by implementing Machine Learning techniques in the Artificial Intelligence space.
The key identified forest risks were – fire, drought, and insects. As forests grow, their ability to capture carbon also grows through the formation of biomass. Large-scale impacts of fire, droughts, or insect outbreaks can extremely reduce biomass, thereby jeopardizing the potential of a sustainable alternative to fossil fuels.
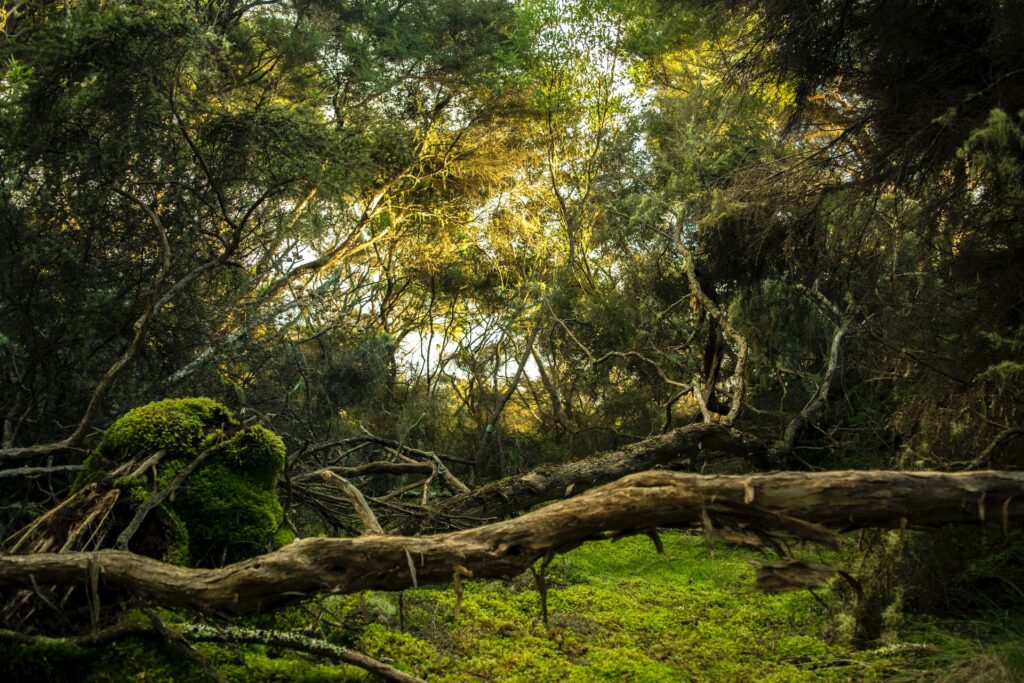
The data used in the Machine Learning model comprised satellite images, ecological data, and hydrologic variables representing water availability.
For the fire risk model, the researchers used fire datasets from 1984-2018 and included fires larger than 202 hectares. Both temporally-varying climatic variables, as well as time-variant vegetation variables, were taken as predictors. The key climatic variables were – temperature, precipitation, and climate water deficit. The vegetation variables – aggregated forest groups, were derived from the National Forest Type Dataset.
They then deployed a ‘hurdle’ regression model (a Regression Analysis in Machine Learning is done to predict a continuous variable (y) based on the value of one or more predictor variables (x)) to predict burn areas as a function of climate and vegetation variables. They combined the linear regression and logistic regression methods in Python using scikit-learn to arrive at the ‘hurdle model’. Scikit-learn is an open-source, robust Machine Learning library in the Python programming language.
The model’s assessment was conducted through R^2 computation between the value derived directly from the data and the model’s prediction (for annual and seasonal trends) and through an area-under-the-ROC-curve (AUC), for the spatial trends.
The fire risk model consistently predicted historical fires (cross-validated AUC: 0.89 – a model whose prediction are 100% correct, has an AUC of 1.0), seasonal patterns (cross-validated R^2: 0.90), and spatial patterns (cross-validated AUC: 0.78).
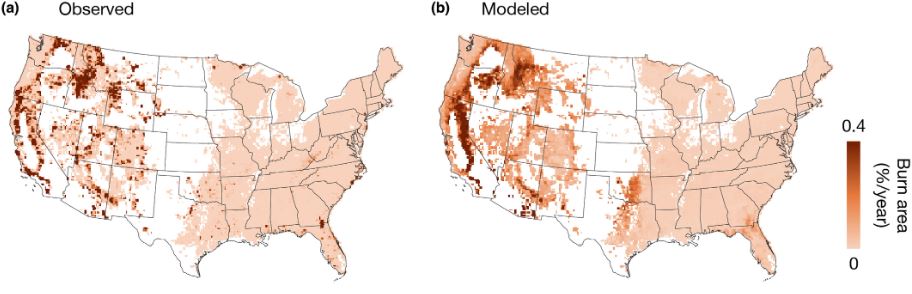
A risk-assessment analysis states that by the end of the 21st century, the fire risk levels are projected to expand throughout the western US, which was historically limited to California and the intermountain western US only.
‘Hurdle’ models for climate-stress and insect-driven tree mortality were developed too. The underlying data was taken from the US Forest Inventory and Analysis dataset. Two generalized linear regression models – a Binomial model with logit link function and a beta-distributed link function were used to represent the ‘hurdle’ model. The logit link function, in statistics, is used to model the probability of success as a function of covariates, i.e., predictor variables. The hurdle model was implemented in R programming language using the ‘glm’ and ‘betareg’ packages.
The climate-stress risk model projected risks with average mortality increasing by a factor of 1.3 in SSP2-4.5, 1.5 in SSP3-7.0, and 1.8 in SSP5-8.5 by 2080-2099.

The insect-risk model indicated increases of 1.2 folds in SSP2-4.5, 1.4 in SSP3-7.0, and 1.7 in SSP5-8.5 by 2080-2099.
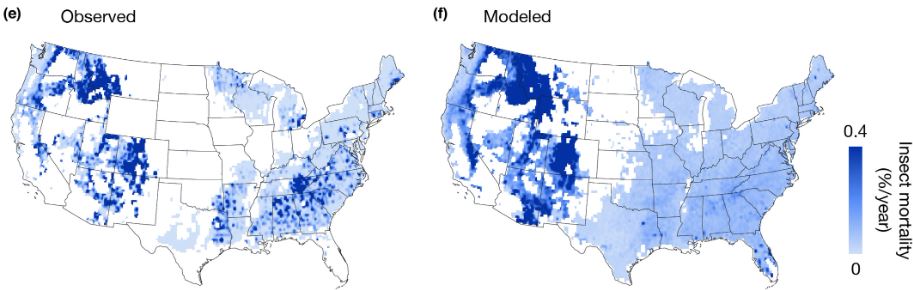
The effects of all three risks – fire, drought, and insect along with biomass can be projected and visualized throughout the 21st century at an interval of 30 years. The warming scenario is considered as ‘medium’ with SSP3.0-7. The color schemes are: Orange denotes Fire, Pink refers to Drought, Blue indicates Insect, and Green denotes Biomass.
Conclusion
The studied climate-sensitive risks to forests have adverse effects on the forest carbon cycle which will eventually disrupt the climate feedback. By leveraging Artificial Intelligence techniques, such as ML methods used in the aforementioned case study, we can arrive at enhanced prediction models and innovative solutions to address environmental challenges, ultimately leading to a sustainable future for all living beings. Forest conservation and policies related to climate change will play an important role in stabilizing the ecosystem as a whole and it is up to governments and citizens alike to take steps toward its restoration.
Notes:
- Future climate risks from stress, insects and fire across US forests
https://doi.org/10.1111/ele.14018 - Forest Risks Map – web mapping tool
https://carbonplan.org/research/forest-risks
Kindly subscribe to our newsletter to be informed about the articles as soon as they are published.
This article is authored by Abhinav Singh.
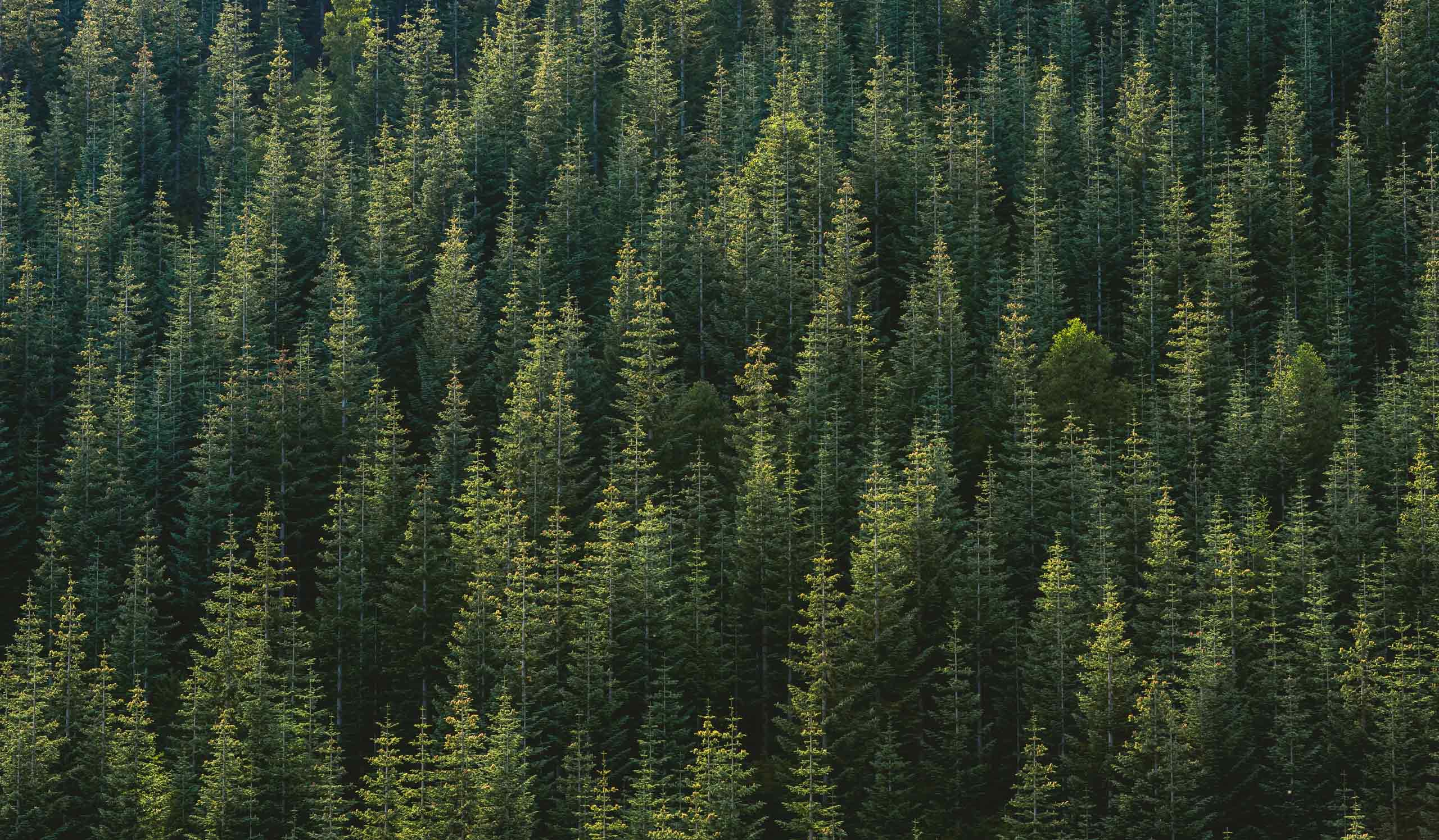
Subscribe to Primitive Proton Newsletter
Sign up to keep up to date with the latest news and curated blogs in the world of space, science, and technology.
Your email is never shared with anyone. You can opt out anytime with a simple click!
WE PRIORITISE PRIVACY.